How to get a grip on data quality
Data quality management is one of those concepts that is hard to define and hard to put into practice.
That is why Jack de Hamer, data management specialist at Type 2 Solutions, has put together a simple 5-step guide with everyday examples to help clarify what data means and how to get a grip on its quality.
Data Quality Management
An online search for the term ‘data quality management’ yields – among others – a link to techopedia.com.
The site defines data quality management as ‘an administration type that incorporates the role establishment, role deployment, policies, responsibilities and processes with regard to the acquisition, maintenance, disposition and distribution of data’.
“In order for a data quality management initiative to succeed, a strong partnership between technology groups and the business is required”, says Jack de Hamer.
‘5 steps to optimal data quality’ presented by Jack de Hamer.
5-Steps towards optimal data quality
Unfortunately, this definition of data quality management does not really offer any suggestions on how to go about it.
That is why we have put together 5 easy-to-understand steps towards optimal data quality.
Step 1. Define the kind of data to be collected and the elements within that data which should be optimized
Step 2. Define rules for each data element and automate the control mechanisms
Step 3. Assign the responsibility for the optimization of a data entity to a single person
Step 4. Automate data validation according to their definition, and keep the results
Step 5. Correct exceptions and adjust their definition if necessary
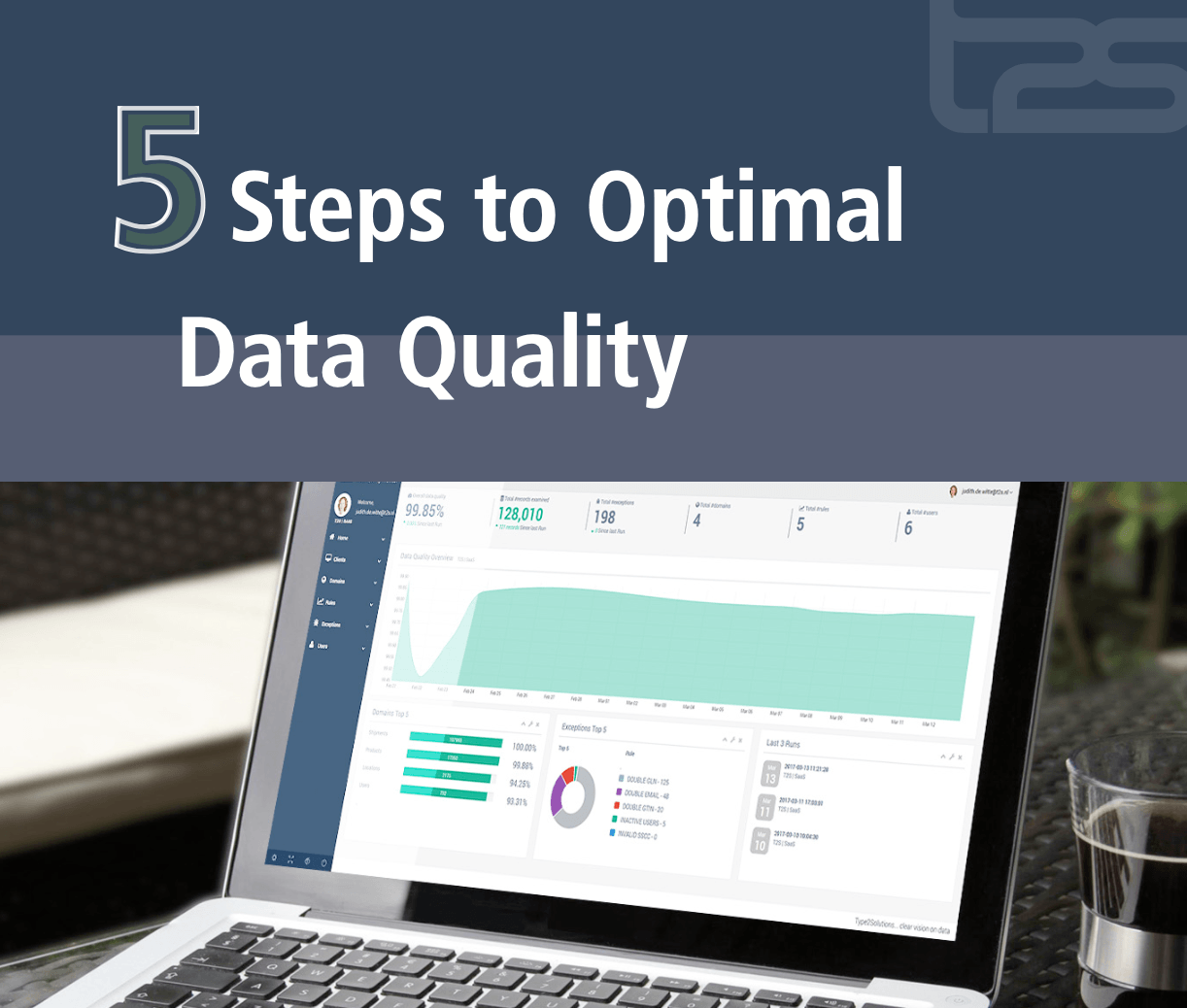
How does it work?
Data is extracted from the data source of an application (step 1). This data is automatically evaluated by the Data Quality Monitor (step 4), according to the business rules resulting from the data definitions (step 2). Exceptions to those business rules are detected, collected, and presented to the data owner (step 3), who can now take appropriate corrective action, either by correcting the data, or by fine-tuning the data definitions.
By repeatedly going through this process, a continuous improvement of the quality of the data, and of the optimization process leading to it, is achieved (step 5).
Data Quality Dashboard
The T2S Data Quality Dashboard helps you to tackle data quality issues. It provides that kind of insight that makes it possible to improve and maintain data quality.
You can experience the Data Quality Dashboard yourself based on an example set with product data.
Start using the Data Quality Dashboard today to see for yourself how it can help you.
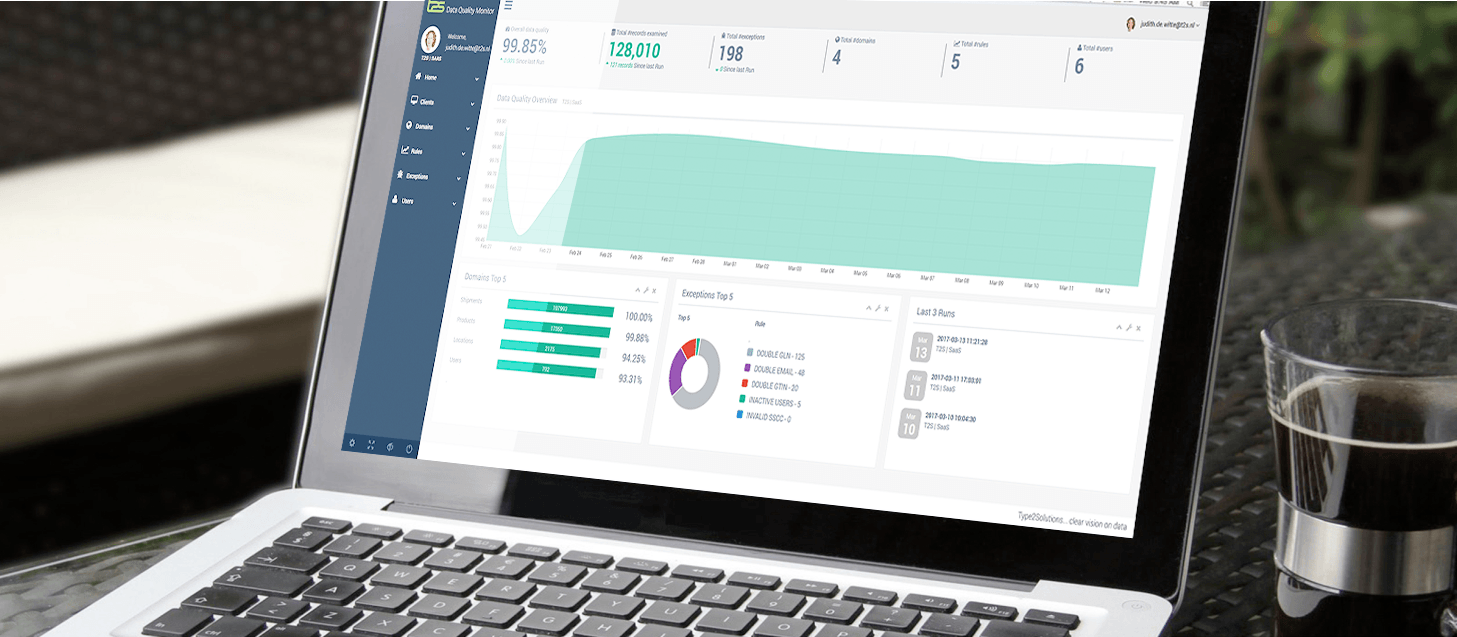
Strategic advantage
It is not easy to put a price tag on ‘poor’ maintenance. According to Jack de Hamer, data specialist at T2S, this is strongly influenced by its consequences.
“The costs can vary from a few euros per customer when mail is returned to thousands or hundreds of thousands of euros in damage as a result of incorrect data on an invoice, customs document or label.”
Read more in the article written by Jack de Hamer about ‘Data quality is a strategic asset‘.
Data quality optimization at Eichholtz
Dennis Hendriks, Director Operations at Eichholtz shares:
“What we started with was quite simple, rather basic; we’ve generated quite a lot of leads through conventions in the past 29 years. This means a large collection of data, data which is far from sanitized. It needed some doing to clean it up.”
Data quality scan
“That’s where Type 2 Solutions provided the answer, by, initially looking broadly at the data and identifying where the mistakes are, removing those mistakes, and enabling us to continue with a clean database.”
Interview with Dennis Hendriks, Operations Director at Eichholtz.
Do you want to improve the quality of your data?
Type 2 Solutions has a lot of experience with the optimization of business data and has supervised various master data management projects. Based on the knowledge gained in practice, we have developed a data quality scan.
The data quality scan helps you on your way and offers an excellent start for any master data management project.
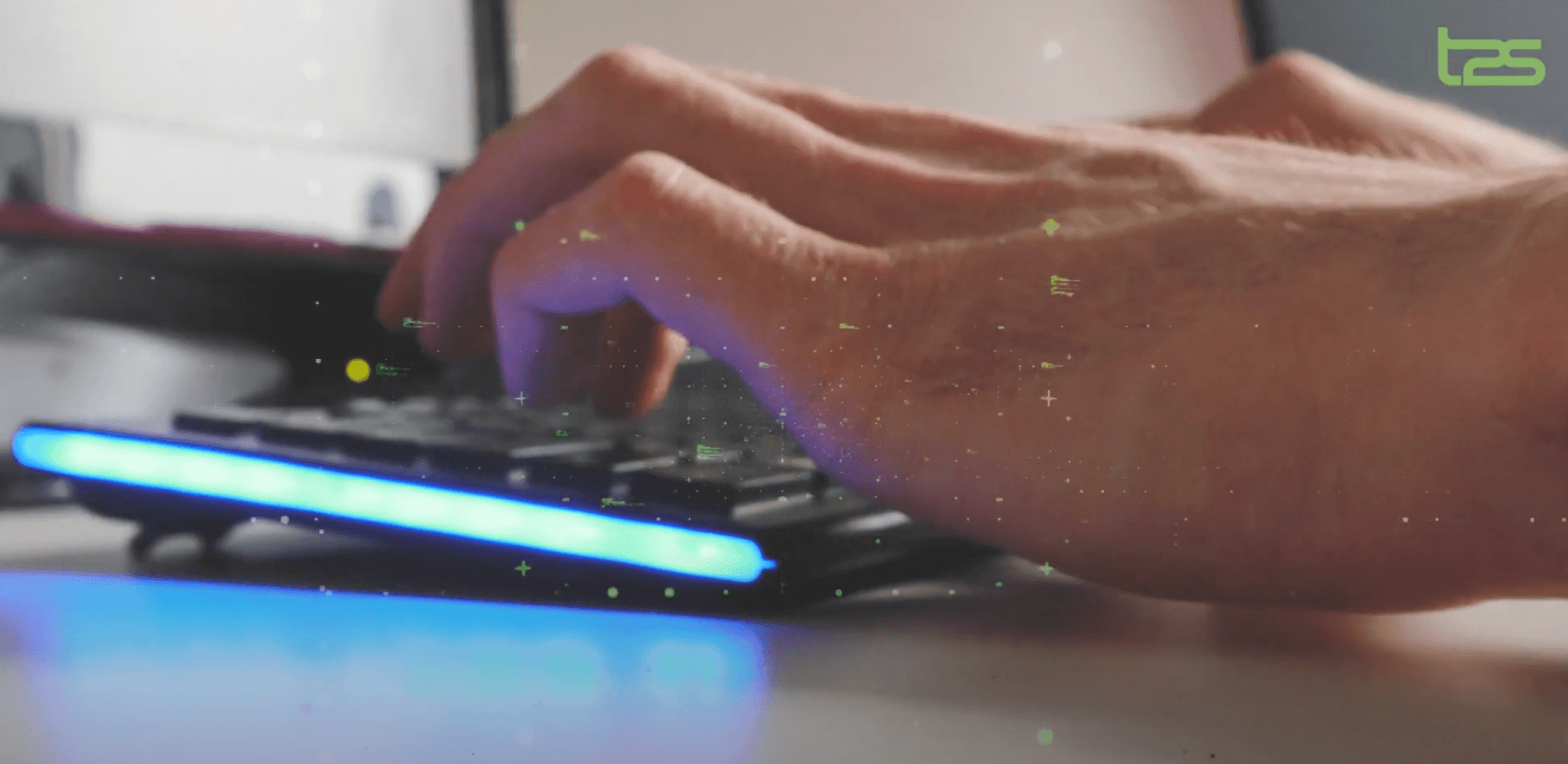